The Power of AI
Is it possible for AI to achieve super- human intelligence?
Prediction of gender using conduction intervals and axis from 12 lead ECG.
Srinivasa D. R. Satti, MD
Introduction:
Artificial intelligence has been used to identify abnormal patterns in electrocardiograms and assist a clinician in making medial diagnosis. These systems have been trained to replicate a human understanding of the data and patterns. Neural networks have been developed to exceed the capabilities of board certified cardiologist in arrhythmia identification from single lead rhythm strips. (Cardiologist-Level Arrhythmia Detection with Convolutional Neural Networks)
In this study, I explore the idea of neural networks capability to extract meaningful information from a 12-lead ECG beyond what a human is capable of. Specifically, the use of a neural network to detect the gender of the patient from the conduction intervals and axis data from the ECG.
Methods
The conduction intervals and axis data was extracted from 93,740 deidentified 12 lead ECGs obtained through the Philips DXL ECG Algorithm in an automated process. This includes the following data points: RR interval, ie heart rate, PR interval, QRS duration, QT and QTc intervals as well as P wave axis, QRS axis and T wave axis. ECGs with missing values were excluded. Thus by this definition, patient in atrial fibrillation or flutter in which a PR interval could not be determined were excluded. The age of the patient was manually entered by the technician obtaining the ECG tracing.
The data points were then normalized by taking the minimum and maximum values for each parameter and:
normalized x = (x-min) /(max-min)
The axis were also standardized to positive values. Thus an axis of -45 deg was encoded as 315 deg.
The gender was recorded as reported by the patient.
Neural Network Architecture
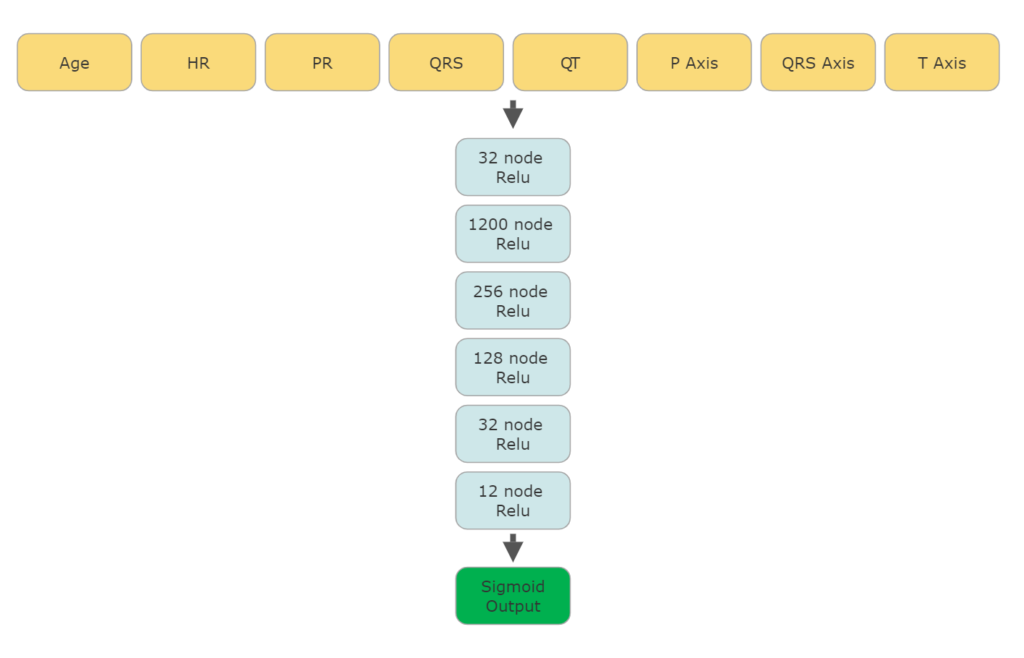
RESULTS
Accuracy was found to be 66.4% overall in predicting the gender. Although this is a low number, this is better than 50/50 change guess that a clinician can offer.
Below is the receiver – operator curve for the neural network.
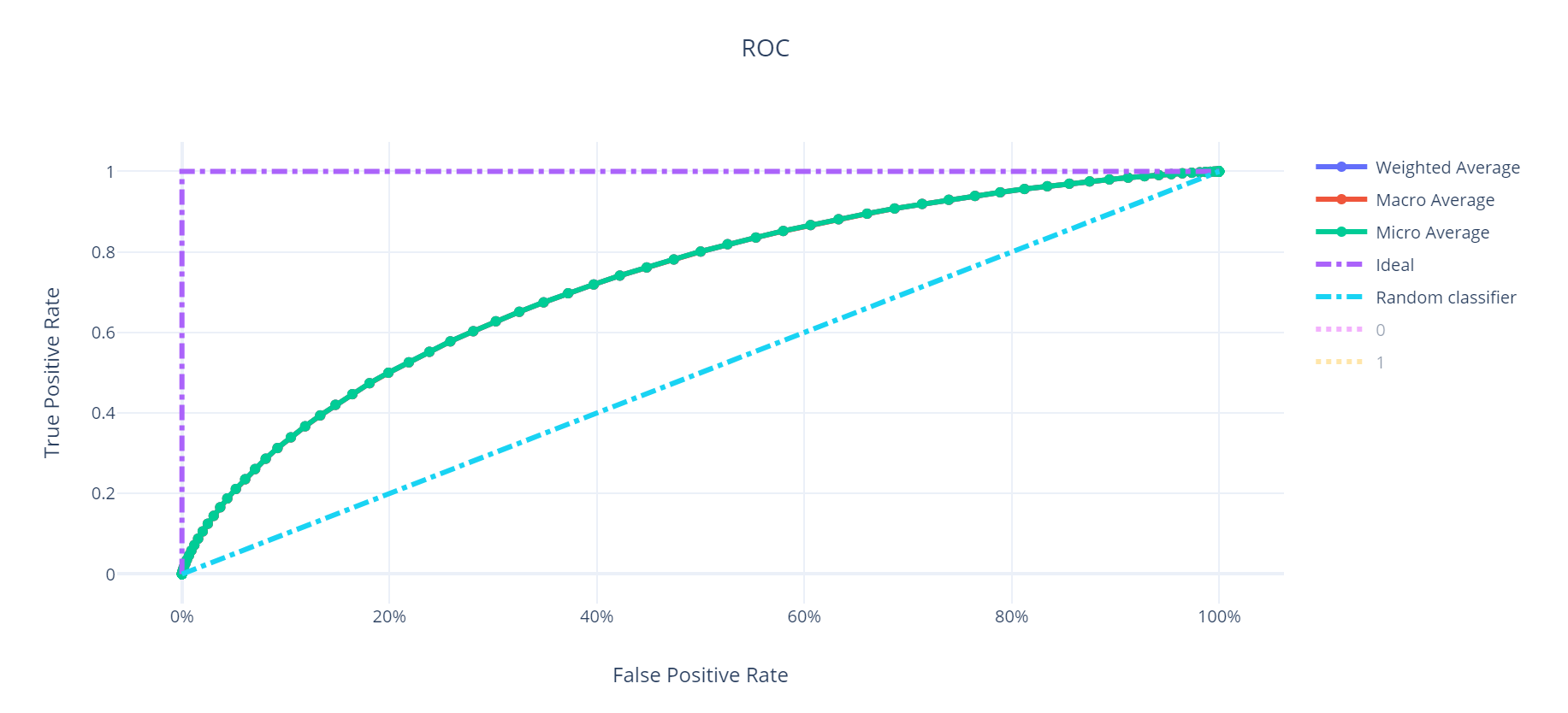
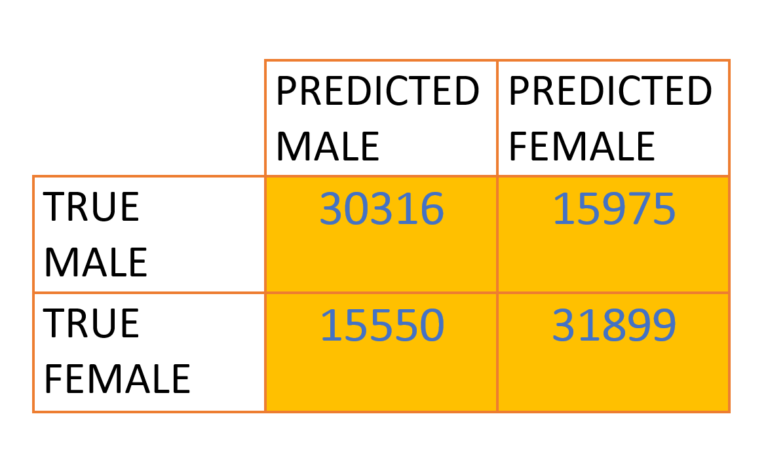
DISCUSSION
The findings from this study shows that gender is predictable from simple parameters of a 12 lead ECG. A trained physician is not able to predict gender based on the same data. This suggests that not only ECGs, but many diagnostic tests may contain useful information that is not apparent to clinicians. The use of artificial neural networks may improve the diagnostic yield of common readily available tests and reduce the need for extensive testing.